Could ChatGPT Make America More Walkable?
No, generative AI shouldn't plan a whole city — but a new study argues it could help identify gaps in our sidewalk networks, tree canopies, and more.
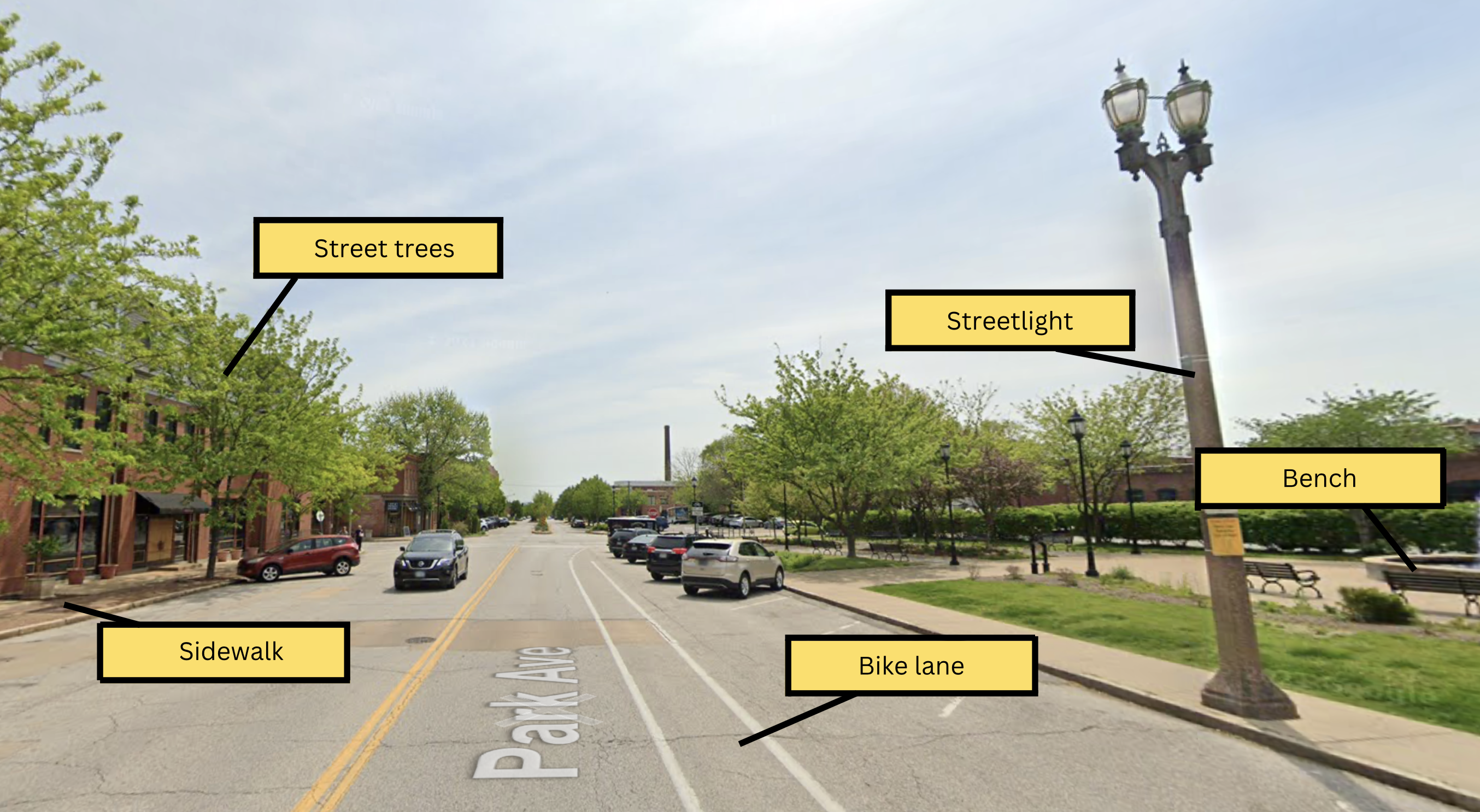
Stay in touch
Sign up for our free newsletter
More from Streetsblog USA
USDOT Secretary Sean Duffy Is Dead Wrong About Bike Lanes
The Secretary of Transportation says he hasn't seen enough data to believe in the benefits of bike lanes. So we put together an explainer help him out — mostly using information from his own department.
Friday Video: Check Out Lorde On a Bike!
The Kiwi singer is on the top of the charts — and in our bike-riding hearts.
Friday’s Headlines Dodge Death
Thankfully, traffic deaths have started to fall. But cars and drivers are still killing too many people. Why?
Friday Video: The High Speed Rail Anthem You’ve Been Waiting For
"Because 220 sure beats going 79."
Breaking: US DOT is Coming For America’s ‘DEI’ Dollars, Threatening ‘Sanctuary’ Cities With Pulled Funds
Advocates are raising the alarm about yet another vague and disturbingly broad letter from Sean Duffy — and the fierce battle that's likely to follow it.